2019 cohort
Meet our 2019 CDT cohort.
Rayna Andreeva
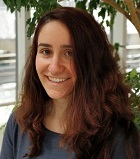
PhD project: Topological data analysis for biomedical images
Supervisors: Rik Sarkar and Miguel O. Bernabeu
Optical coherence tomography angiography (OCTA) imaging is a relatively new modality for the discovery of retinal biomarkers (changes which are potential source of measurable indicators for particular disease) linked to chronic diseases. Several studies have already shown the potential of using hand-crafted geometric features on OCTA images for identifying patient status. However, they depend on the manual segmentation and pre-processing steps, which are prone to errors. In contrast to previous work, we suggest the usage of topological information which does not depend on geometric calculations, is more robust to noise, works well with small datasets and it is interpretable.
We aim to investigate whether features based on topological (shape) information can help in identifying patient status from a relatively small dataset. Second, we plan to achieve interpretability, which is understanding why certain decisions or predictions have been made (which is particularly useful in clinical setting) and to discover novel topological biomarkers. Further, we plan to extend this work to other datasets and applications, such as MRI scans of tumors as shape and connectivity are also vital in that domain.
Nikitas Angeletos Chrysaitis
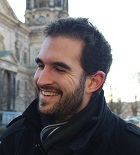
PhD project: Examining and extending Bayesian models of Autism
Supervisors: Peggy Series, Renaud Jardri and Stephen Lawrie
In order to deal with the noise of the environment and imperfections in our sensory organs, our brain combines sensory inputs with previous knowledge of its environment. This framework has provided deep insights into the workings of the brain and has also offered promising hypotheses on the nature and causes of psychiatric disorders, such as autism. Usually, autistic individuals are assumed to rely more on information from their senses compared to previous knowledge. However, we still do not know the exact details of this imbalance, as the relevant studies do not rely on a common methodological approach, making difficult to synthesise their findings. During this project, I will examine and attempt to resolve central disputes within this field. To do that, I will rely on a thorough analysis of the methodologies and findings of past studies, as well as the replication of their designs and extension of their computational methods to account for the large variety in their findings.
Matúš Falis
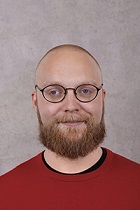
PhD project: Addressing Concept Sparsity in Medical Text with Medical Ontologies
Supervisors: Beatrice Alex, Alexandra Birch-Mayne and Will Whiteley
One of the major issues in machine learning in the medical domain is the existence of a large variety of concepts (diseases, procedures, etc.), some of which are very rare. Due to the rareness of certain diseases, many hospitals may have never had a patient with a specific rare disorder, or never had certain procedures performed on a patient. Consequently, these rare concepts appear in clinical text less frequently. Furthermore, new concepts enter medical knowledge, such as new treatment approaches, procedures. A machine learning model trained on a dataset will find it problematic to address these previously unseen concepts. This issue becomes a major problem if these potentially previously unseen concepts are the labels the model is supposed to predict, such as labelling medical text (e.g., hospital discharge summaries) to indicate what diseases the patient had.
Medical knowledge is recorded in a structured format in medical knowledge bases or ontologies, such as UMLS, ICD, or SNOMED-CT. Ontologies include information on disorders regardless of how frequently they occur. Furthermore, ontologies are updated on a regular basis with new findings in the medical field. Ontologies differ in structure and the purpose they are used for. Medical text is usually labelled with the use of only as single ontology that best fits the setting. Furthermore, some ontologies are common across different languages and medical systems with only minor differences in localised versions.
The goal of this thesis is to explore means of integrating medical background knowledge from secondary corpora that differ either in the ontology used for labelling or the language of the corpus in order to enhance the representation of the concepts in the primary ontology used in the primary task. Our focus will be on deep learning models. We are particularly interested in concepts that are either infrequent or absent in the training data.
Alessandro Fontanella
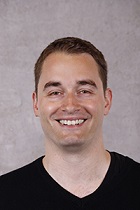
PhD project: Deep learning methods to identify ischemic stroke lesions from CT scans of the brain
Supervisors: Amos Storkey and Grant Mair
An ischaemic stroke happens when a blood clot cuts off the blood supply to the brain. A computerized tomography scan, which uses rotating X-ray machines to create crosssectional images of the body, is often used for diagnosing this disease. Automatic detection of the lesions cause by ischaemic stroke trough a computer model is an ongoing area of research, since it could save time and resources. Most previous studies aiming at this goal focused on statistical methods, while we propose to use deep neural networks, artificial neural networks with multiple layers. In previous works, the authors usually had to manually select features from the images to be used as input for the model. On the other hand, with our approach we could directly give the images as input to our models, that are able to extract features automatically. An analysis of which features are picked up by the network can be performed a posteriori, for interpretability reasons. By collaborating with clinicians, we could work towards the goal of adoption into clinical practice of our model.
Domas Linkevicius
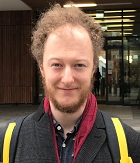
PhD project: Supplementing models of synaptic plasticity with machine learning
Supervisors: David Sterratt, Melanie Stefan and Angus Chadwick
The human brain is an extremely complex structure, underpinning our cognitive functions, such as speaking, memory, attention and others. One of the phenomena that allows the brain to support all of these functions is its plasticity – the networks of neurons can change and respond to external stimulation in adaptive ways. One of the ways in which the networks of neurons can change is by changing the connection strengths between the cells by a process called synaptic plasticity. Synaptic plasticity is an intricate process that involves thousands of different molecules and is not yet fully understood.
There have been many attempts to create computational models of synaptic plasticity. However, all of them have included only small parts of the complex biochemical network present in synapses. The main reason is that there simply is not enough data about this biochemical network, prohibiting any attempts to simulate synaptic plasticity at a detailed level. In this PhD project we propose a way to bypass this challenge by using machine learning. Machine learning algorithms use data to learn models of various functions, such as face recognition or language translation, in a principled and automated manner. We would use machine learning to model the output activity of the part of the synaptic biochemical network that is poorly understood. This output activity would affect the well-known part of the biochemical network, helping to reproduce existing experimental studies. The resulting model would be helpful in investigating and understanding various neurological illnesses.
Evgenii Lobzaev
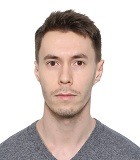
PhD project: AI-driven design of enzyme replacement therapies
Supervisors: Giovanni Stracquadanio and Dominic Campopiano
Enzymes are proteins that accelerate chemical reactions. They are needed to act upon certain molecules, called substrates in order to produce other molecules, called products. As an example, mutations in GBA gene lead to accumulation of a particular lipid, glucocerebroside, in cells and certain organs. This happens because GBA gene mutations cause deficiency of the enzyme glucocerebrosidase, which acts on glucocerebroside. Such condition is known as Gaucher’s disease and is treated by injecting a deficient enzyme into the patient.
It is usually ineffective to simply inject wild-type human enzyme because of enzymes loose activity in blood and an adverse immune response for the patients. Therefore, human enzymes must be carefully engineered in order to be suitable for disease’s treatment.
While it is possible to design small peptides with standard approaches such as directed evolution (DE), it is not feasible (both in terms of time and money) to use them for long proteins, such as enzymes. Generative Machine Learning (ML) offers an alternative approach to designing new enzymes. Recently, conditional recurrent neural networks (cRNN) were successfully used to generate novel antimicrobial peptides and protein structures, but scaling these methods to design complex molecules, including enzymes, remains a largely unexplored field.
We plan to use deep generative models for enzyme engineering. We will integrate and use different sources of publicly available biological data as an input to our model and use advanced ML approaches to train the model. The result of the model will be enzymes that satisfy the required properties.
Emanuela Molinari
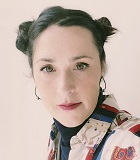
PhD project: Health, mental health, social and economic outcomes in survivors of childhood cancer
Supervisors: Valerio Restocchi, Peter Hall and Claire Niedzwiedz
Based on the data of 235,435 survivors of cancer diagnosed when young, recent studies have shown a substantial 5-fold excess of death during adult life when compared to the expected survival from the general population (30% versus 6% death rate respectively). The second most common cause of the excess of number of deaths is caused by circulatory disease. Among studies on cancer diagnosed when young, none has looked at mental health long-term effects of survivors. Two UK-wide research priority setting projects overseen by the James Lind Alliance, defined in the top 10 UK priorities for cancer survivors, their families and their healthcare professionals. Almost half of them concerned the long-term effects of a cancer diagnosis and treatment at young age, the importance of finding a way to compare treatments on the late-effects and developing targeted follow-up care to prevent serious health consequences. The project responds to the increasing demand from government bodies, patients and carers on quantifying the impact of cancer survivorship, exploiting the NHS investment in electronic health data and the UK drive in developing machine learning (ML)-tools for the healthcare. We will analyse big data of cancer registries linked with routine NHS data and public sector data through the National Safe Haven analytic platform for anonymised extracts of data (electronic Data Research and Innovation Service - eDRIS). We will use standard statistical approach and ML tools for better prediction of cancer outcomes.
Michael Stam
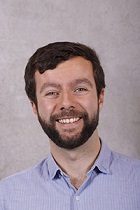
PhD project: Machine learning for reliable protein design
Supervisors: Chris Wood, Diego Oyarzún and Nadanai Laohakunakorn
Proteins are tiny molecular machines that are critical for life to exist. They have a large variety of roles such as protecting the body from bacteria and viruses, carrying messages from different parts of the body and even creating different types of materials. Amino acids are the building blocks that are combined to create proteins. Currently, nature has only explored a small percentage of the different ways that these building blocks can be put together. This means that there is an opportunity to explore different methods to combine these amino acids and to create new proteins. These proteins can be designed for different applications in medicine, agriculture, energy and other areas. However, this is a really challenging task and many designs fail when tested in labs. Therefore, this PhD project aims to understand the main reasons why these designs fail, and to use this understanding to improve the design process. First, experiments will be performed on designed proteins to allow us to collect information about the reasons they succeed or fail. We will then use this information, combined with existing knowledge of proteins, to develop machine learning methods to help improve the reliability and reduce the cost of the protein design process. These improvements could have a huge impact, as they could make it easier for scientists to develop new proteins, to tackle challenges we face in areas such as medicine, agriculture and energy.
Natalia Szlachetka
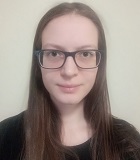
PhD project: A data-driven approach to engineering a con-ikot-ikot toxin-based fluorescent probe for super-resolution imaging of AMPA receptors
Supervisors: Jelena Baranovic and Chris Wood
Dementia is a term for a group of symptoms related to loss of brain function (such as memory, thinking, and behaviour). According to the WHO, it affects around 50 million people worldwide. Understanding how proteins called AMPA receptors move into and out of the synapses (sites where neurons meet) can help us understand the mechanisms of some forms of dementia. To do this, movements of AMPAR can be studied by attaching fluorescent labels to individual receptors and following them under a microscope. Currently available labels are often bulky and prevent AMPAR from moving in a way they would normally, without a label present. In this project, a small toxin found in the venom of a sea snail Conus striatus will be used to design a compact fluorescent label that would bind to AMPA receptors. The structure of the toxin, called con-ikot-ikot, will serve as a skeleton for protein designs proposed by computational methods. Simulations involving these designs will be carried out to determine their usefulness for the purpose of fluorescently labelling AMPA receptors. The most successful proposed proteins will be selected to be produced in the lab. They will then be used in experiments to develop fluorescent labels for tracking AMPA receptors under the microscope to study their movements in health and disease.
Katarzyna Szymaniak
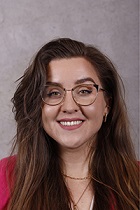
PhD project: Co-adaptive Human-Machine Learning
Supervisors: Kianoush Nazarpour, Agamemnon Krasoulis and Timothy Hospedales
Prosthetic limbs can enable people with limb differences to regain their independence and return to work. Research on upper-limb prosthetic limbs aims to replicate the functionality given by our biological hands. Despite the advancement in prosthetic technologies, about 40% of amputees rejects their devices, citing the lack of function and individualised training as the key reasons. The goal of this PhD project is to enhance the function of upper-limb prostheses with advanced machine learning; informed with studies of human sensory-motor adaptation. Specifically, for the first time, the theoretical framework of active learning will be explored within the context of prosthesis control; allowing the human user to remain and shape effectively the control loop. Such an approach will enable the so-called, but yet unachieved objective of user-prosthesis co-adaptation and will lead to truly personalised prosthetics. This PhD project will begin with the adaptation of existing active learning frameworks for prosthetics control to understand better the limitations and opportunities. Further, the work will entail the development of novel theoretical and experimental paradigms to test the approach within the laboratory and in a real-life setting.